How Does Conversational AI Address the Limitations of Insurance Support Chatbots?
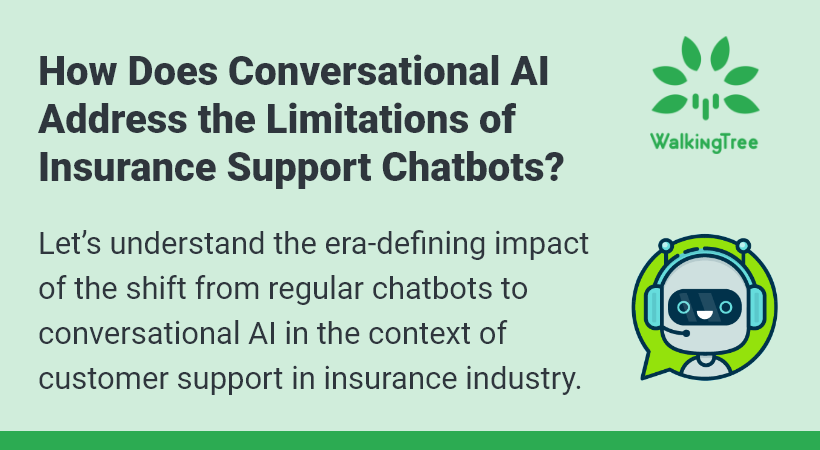
Insurance demands personalization more than any other financial domain since the risks covered by insurance policies depend on a plethora of customer-specific or object-specific factors. Just because insurance is such a hyper-personalized domain, one-on-one communication, and individual risk and requirement assessment play such an important role for insurance products and the customer experience.
How insurance products are designed, advised, sold, and marketed has evolved over the years. The increasing risk factors and their characteristics made it obvious for insurance companies to focus on more personalized services than ever before. This is where conversational AI opened new opportunities for insurance companies to streamline their customer support, without compromising on a personalized experience for the customers.
There are examples galore in the insurance industry where conversational AI is doing a great job of resolving customer queries faster. But there are also examples around erroneous responses or inability to incorporate brand voice. As the uses of cutting-edge conversational AI technologies are just at their budding stage and still most customer-support chatbots mostly rely on prefigured decision-tree for answering queries, these shortcomings should be viewed as part and parcel of the overall customer support experience.
But conversational AI technologies and tools are ripe enough to help insurance support chatbots leap here. How conversational AI can address the flaws and fault lines that are common with many of the customer support bots used by insurance companies? Let’s find out.
Inability to address deep-probing queries
It is common for traditional chatbots powered by preconfigured decision-tree to be able to answer only a set of queries. However vast and extensive a decision tree is made to cover as many user queries as possible, for the insurance domain where endless queries can come out of a labyrinthine maze of variables, it is bound to fall short whenever customers probe a little deeper.
For example, when a customer asks a bot about your eligibility for a policy or about the premium calculation based upon the risk factors, it can just give precise and helpful answers. But by going a step further when the customer asks the same bot to compare a few different policies and their differences on specific features like risk coverage, eligibility, and cost of insurance, the same bot may not give a clear picture to help the customer take a decision.
On the other hand, conversational AI can ensure proactive answering capabilities as it can update itself from time to time with new intents and corresponding information. Even then, there are use cases of conversational AI that still lack abilities to address customer queries in-depth. This happens because conversational AI still needs one vital type of human intervention in the way of training the data model with multiple intents and variations of frequently used phrases and expressions.
The ability to communicate in natural and everyday language and wider coverage of customer intents are two areas where conversational AI can play an impressive role to deck up customer support capabilities. But to make the most of these capabilities, continuous training on multiple customer intents and spoken or written expressions remain imperative.
The problem of multiple segment-specific support chatbots
Many chatbots are built in a segregated manner to focus on a set of customer issues for a particular channel such as sales, onboarding, documentation, claim processing, grievance, complaints handling, etc. Since insurance is a multifaceted domain, multiple chatbots undermine the seamless customer experience. This happens mainly with traditional rule-based chatbots as their horizon of capabilities is fixed by the set of queries branched out from a decision tree.
Customers making queries on policies may end up asking about the rate of claim settlement or the intricacies of claim processing. Such cross-segment queries are common in customer communication and it needs the comprehensive approach of conversational AI.
A seamless and consistent conversational experience across the channels is still something the automated support mechanisms in most insurance companies lack. There are also concerns regarding flagging an unresolved query for live support. How well and smoothly this is done can have a grave impact on the customer experience.
Lack of brand voice
Chatbots representing a customer support channel are often viewed as virtual extensions of business brands. Naturally, chatbots from the aspects corresponding to the look and feel to the tone of the conversation should represent the brand voice. Several chatbots across industries including that of insurance, lack this branding element.
Conversational AI with its ability to communicate in natural language does not just rely on the look and feel of the interface for bringing the brand before the customers. It can progressively show its ability to incorporate unique tones and expressions associated with an insurance brand. While proactive learning capability and frequent training of data models are standard attributes of conversational AI, they can open up new ways to shape the brand voice in communication.
Lack of personalization and inclusivity in communication
Humans, irrespective of the circumstances, always expect their concerns to be addressed humanely, i.e., with expressions and phrases of everyday communication they are habituated with. Though in the back of their minds people know they are conversing with a bot, they still adore and expect the ‘make-believe’ world of natural dialogue. But there, most bots disappoint at a certain point.
The personalized communication of conversational AI is much in focus because of its ability to incorporate natural and everyday linguistic expressions and phrases. With an inclusive approach to incorporate linguistic variables, conversational AI can create the much-appreciated “make-believe” world of conversing with a bot that understands the customers personally.
The usual disappointment of regular support-chatbot communication in anticipating individual concerns and addressing personal ways of putting a query can now be mediated by the Natural Language Generation (NLG) models of conversational AI. Since continuous training of data models to cover a wider variety of intents, variations of conversational paths, and corresponding phrases, expressions and words are imperative for conversational AI, customers can expect more tailored communication specific to their queries.
Looking Forward
It is heartwarming to see how insurance companies are gearing up to make the promises of conversational AI come true for customer support, especially when the technology is fast evolving and discovering enhanced capabilities. As we explained, traditional Chatbot support is already outdated making room for conversational AI to take over. To know how customer experience in the insurance sector is taken to the next level through conversational AI, read our next blog here.
Join Scott, Abhilasha, and Shubham to have an elaborate understanding of the topic on our next webinar on 3rd November 2022: Conversational AI in Insurance: Challenges & Opportunities. To register for the webinar, click here.